报告题目:Transfer learning with high-dimensional convoluted rank regression
报 告 人:张日权 教授、博士生导师
上海对外经贸大学统计与信息学院
报告摘要:Transfer learning can leverage information from the source domain to improve the estimation or prediction accuracy of the target task. For the high-dimensional linear regression model with sub-Gaussian noise, so-called Trans-Lasso algorithm has been proposed to boost the learning performance on the target domain. However, such algorithm may not lead to efficient estimates when the errors are heavy-tailed. In this paper, we investigate the penalized convoluted rank regression (CRR) under the transfer learning framework, aiming to provide robust estimators when dealing with heavy-tailed noise. The convolution smoothing technique improves the smoothness of the loss function without introducing any bias. In the high-dimensional setting, we first propose a transfer learning algorithm on the penalized CRR models with known transferable sources, and establish l2/l1-estimation error bounds for the corresponding estimators. Besides, we propose a transferable detection method to select informative sources and also verify its consistency. At last, we provide extensive numerical simulations and real data analysis to demonstrate the effectiveness of our proposed methods.
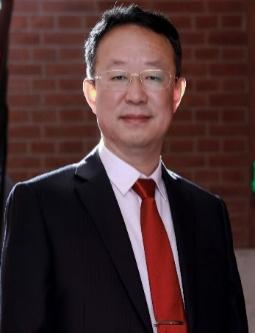
报告人简介:张日权,上海对外经贸大学统计与信息学院院长,教授、博士生导师;中国现场统计研究会大数据统计分会理事长;上海统计学会副会长;中国商业统计学会数据科学与商业智能分会副理事长;中国现场统计研究会高维数据统计分会副理事长;中国现场统计研究会经济与金融统计分会副理事长。《统计研究》《数理统计与管理》《统计学报》、Statistical Theory and Related Fields编委;《应用概率统计》期刊第八届、第九届副主编。教育部统计与数据科学前沿理论及应用重点实验室创始人;曾任华东师范大学统计学院院长,教育部统计与数据科学前沿理论及应用重点实验室主任。主要从事大数据统计,金融统计,半参数统计,高维数据分析,函数型数据分析、统计机器学习研究;主持多项国家自然科学基金、上海市科委重点项目等20多项;在国内外权威期刊发表学术论文200余篇,出版专著3部,教材4部(其中两本是国家级规划教材);获得上海市育才奖、上海市自然科学奖、上海市教学成果奖等奖励或荣誉称号20多项。
报告时间:2023年11月6日下午14:00-16:00
报告地点:腾讯会议103-842-145
联 系 人:祝志川
欢迎老师和同学参加!